Sponsors: InCoB 2012 and ICIW 2012
Prediction task:
Predict peptides naturally processed by MHC Class I pathway ("eluted peptides") for each target MHC molecule. For each target molecule, the competitors are asked to submit a set of predicted eluted peptides from the test set.
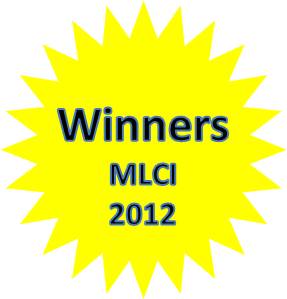 |
A total of 32 submissions were submitted for the competition. Of these, 24 submissions (Group 1) provided a set of thresholds (elution score based predictors) for each peptide and each MHC molecule. Another 8 submissions (Group 2) provided lists of peptides that were predicted as eluted from specific MHC molecules (eluted peptide list based predictors) for each of 8 studied MHC alleles. The NetMHC 3.2 server (1D-BENCH) results were used as a benchmark method. |
Winning Team | Predictor No. | Prediction Method | Winning Category |
Lundegaard C, Lamberth K, Harndahl M, Buus S, Lund O, Nielsen M, Technical University of Denmark | 1D-BENCH | NetMHC 3.2 (Reference) | Group 1: A*0201 |
Giguere S, Drouin A, Lacoste A, Laval University, Canada | 2F | A Bayesian model averaging method over several SVMs using the GS kernel. | Group 1: B*0702, H-2Db, and H-2Kb |
Nielsen M, et al., Technical University of Denmark | 9D | A combination of NetMHC, NetMHCpan and MHCkernel predictions. | Group 1: B*3501 and B*4403 |
Giguere S, Drouin A, Lacoste A, Laval University, Canada | 2D | A SVM classifier and a novel string kernel (GS kernel). | Group 1: B*5301 |
Xiang Z, He Y, University of Michigan Medical School, Ann Arbor, MI, USA. | 20D | A position-specific scoring matrix (PSSM) with statistical P-value as the cutoff. | Group 1: B*5701 |
Yu Ting Wei, Department of Probability and Statistics, School of Mathematical Sciences, Peking University; Wen Jun Shen and Hau-San Wong, Department of Computer Science, City University of Hong Kong | 14A | ConsMHC: a consensus program incorporating the results of kernelRLSpan-I, NetMHC, NetMHCpan and PickPocket by SVM | Group 2 |
Click for the test data sets with elution properties included
Data:
Target MHC molecules:
- Human: HLA-A*0201, -B*0702, -B*3501, -B*4403, -B*5301, and -B*5701
- Mouse: H2-Db and H2-Kb
Peptides:
- Eluted peptides for each target molecule include 8 to 11mers
- For each MHC molecule, a set of 8 to 11-mers is given for all data sets
Training sets: click to access them
- Eluted peptides from target MHC molecules
- Binding peptides for target MHC molecules
- Non-binding peptides target MHC molecules
Test set: click to access it
A composite set of 18,199 test peptides is given (6241 8-mers, 5190 9-mers, 3537 10-mers, and 3231 11-mers). Test sets for individual target molecules are embedded within the composite data set.
FAQ
- We are provided two binding peptide datasets (eluted and binding peptides). Taking into account that eluted peptides are binding peptides, is it correct to consider all peptides in the binding dataset as non-eluted?
show/hide the answer
No, binders can be in the either class - eluted or non-eluted.
Assumptions:
- A set of eluted peptides for each MHC molecule is a proper subset of binding peptides for that MHC molecule. A proper subset does not include all members of the set that it belongs to. The set of binders, therefore, will contain both eluted and non-eluted peptides.
- For a given MHC molecule, an eluted peptide must be a binder to a given MHC molecule.
- A non-binders cannot be eluted peptides.
- Any two MHC molecules will have distinct, and in some cases overlapping, sets of eluted peptides, binders, and non-binders as compared to another MHC molecule.
- I noticed that the peptides in the test set are not categorized into alleles. Should we apply each allele-specific predictor to the entire test set? I am afraid that the data will be evenly unbalanced.
show/hide the answer
- Yes, each allele specific predictor should be applied to the entire test set.
- Balancing of test sets is not an issue here because the scoring formula is not sensitive to the proportion of positives and negatives in the test set.
- What is the format for submitting the predictions? For each test peptide, should we predict whether it is Eluted or not? Should we predict the more likely label among the three labels (Eluted, Binder, and non-binder)?
show/hide the answer
The prediction task: For each target molecule (variant), the competitors are asked to submit a set of predicted eluted peptides from the test set (see Q4). This set, predicted eluted set will be assigned label "Eluted". By default, all other peptides in the test set will be predicted not eluted peptides and will get label "Not eluted". These lists will be mapped to the actual test set for each target molecule.
The format:
A*0201 | Allele |
Predicted A*0201 positive 1 | PEPTIDE1 |
Predicted A*0201 positive 2 | PEPTIDE2 |
... | ... |
Predicted A*0201 positive N | PEPTIDEN |
- What is the performance metric for evaluating the predictions?
show/hide the answer
The performance metrics will be based on maximizing the formula:
- At the last competition, it was only possible for each registered person to make submissions for one method. Since we would like to use the competition as a benchmark of a broad panel of methods, we would like to have individual persons submitting predictions for multiple methods. Would this be possible?
show/hide the answer
This question addresses a key issue. On one hand it is reasonable to allow individuals to submit multiple predictions based on different methods. Furthermore, if a particular predictor provides threshold-based classification it is reasonable that the results for several thresholds are submitted. On the other hand, the competition should discourage "shotgun approach" i.e. the hasty use of a wide range of techniques. Subject to a discussion and approval by the MLI 2012 Committee, the following rules will be allowed:
- Up to three submissions representing essentially different method will be allowed per participant for each molecule (subject to approval). The participants will need to demonstrate that these methods are sufficiently different.
- Up to three submissions representing the same method, but different prediction thresholds will be allowed per participant for each module (subject to approval)
- It is clear that they are eluted peptides, but it is not clear from what cell line, and if they all originate from self-proteins. The latter point is essential since most (if not all) prediction methods for antigen processing rely on some degree of context beyond the actual ligand sequence in order to predict cleavage and TAP transport.
show/hide the answer
This is an important point. The goal of this competition is to clarify or improve our knowledge about naturally processed peptides and experimental methods used to identify them. Naturally processed peptides are the result of several processing steps and include multiple processing pathways. Most, if not all, of these pathways are not described or modeled in sufficient detail to enable significant insights into identification of high-quality vaccine candidate peptides. Key questions, assuming the accuracy of experimental methods (HPLC + Mass Spectrometry), addressed by this competition are:
- Can we predict naturally processed peptides, i.e. eluted peptides, from the peptide sequence alone, i.e. without knowing the flanking sequences? To what extent are these predictions possible?
- How much can prediction of naturally processed peptides be improved if we use tools as a reference?
- Can we identify non-eluted peptides by identifying patterns from core sequence of the peptides?
- To what extent the information contained in eluted peptides can be used to minimize the number of false positives?
- Finally the test data do not have associated MHC restrictions. Are we right in assuming that the task of the prediction will hence be to identify the most likely MHC restriction element of the 8 molecules included in the benchmark using prediction methods? However if this is the case, it is not clear to us which performance measure will be used to evaluate the different predictions.
show/hide the answer
Associated MHC restrictions are not stated. This will make prediction task and submission easier for the participants. See figure at Q3.
Publication:
Winning algorithms and associated papers will be invited to be submitted for publication in the Journal of Immunological Methods (subject to peer review).
Background
Experimental studies of immune system and related applications such as vaccine design, optimization of therapies deal with high combinatorial complexity. The experimental approaches in such fields are time-consuming and expensive. More efficient approaches involve pre-screening by computational models, followed by experimental validation using selected key experiments.
Peptides that are presented by Major Histocompatibility Complex Molecules (MHC) are important targets in studies of cell-mediated immunity, regulation of immune responses, vaccine research, and transplant rejection. Naturally processed MHC peptides are usually identified by fractionation (e.g. using HPLC) followed by mass spectrometry measurements. We refer to these peptides as "eluted". These peptides are important targets in studies of cell-mediated immunity, regulation of immune responses, vaccine research, and transplant rejection. We will deal with MHC Class I molecules in this competition.
Eluted peptides have several properties. It is widely accepted that they must (link to picture):
- be processed - cleaved, transported, and loaded onto MHC
- bind to an MHC molecule
- maintain stable association with MHC molecule
A number of computational models have been developed for prediction of MHC-binding peptides (MHC ligands) and standards for their assessments have been developed (Zhang et al., 2011). MHC-binding peptides are normally determined using biochemical binding assays. A subset of naturally processed peptides comprising of estimated 5-15% of MHC-binding peptides are considered as useful targets for vaccine development. In silico identification of candidate MHC-binders is a standard methodology in screening targets of immune responses and vaccine targets. Some of algorithms for in silico prediction of peptide binding are highly accurate, while others need improvement. Algorithms that can accurately identify naturally-processed MHC-binding peptides would further reduce cost of target validation by 5-10 fold. Machine learning-based methods offer a great promise for further advancement of prediction systems in this field.
The purpose of this competition is the development and the assessment of computational methods for prediction of eluted peptides (naturally processed peptides). Comparison of results with unpublished experimental data will help determine best performing algorithms. In addition, this competition will bring together researchers from molecular biology, immunology, bioinformatics, computer science, statistics, and other related field with the ultimate goal to expand the set of tools available for study of immunology and vaccinology.
Training sets:
- HLA-A*0201 datasets
- Eluted peptide dataset: 1370 eluted peptides
The lengthes of the 1370 peptides vary from 8 to 11. The numbers of 8-mer, 9-mer, 10-mer, and 11-mer peptides are 22, 971, 258, and 119 respectively.
- Binding peptide dataset: 5625 binding peptides
The lengthes of the 5625 peptides vary from 8 to 11. The numbers of 8-mer, 9-mer, 10-mer, and 11-mer peptides are 34, 4244, 1278, and 69 respectively.
- Non-binding peptide dataset: 6127 non-binding peptides
The lengthes of the 6127 peptides vary from 8 to 11. The numbers of 8-mer, 9-mer, 10-mer, and 11-mer peptides are 29, 4842, 1213, and 43 respectively.
- HLA-B*0702 datasets
- Eluted peptidedataset: 170 naturally processed peptides
The lengthes of the 170 peptides vary from 8 to 11. The numbers of 8-mer, 9-mer, 10-mer, and 11-mer peptides are 7, 122, 28, and 13 respectively.
- Binding peptide dataset: 1197 binding peptides
The lengthes of the 1197 peptides vary from 8 to 11. The numbers of 8-mer, 9-mer, 10-mer, and 11-mer peptides are 13, 1032, 143, and 9 respectively.
- Non-binding peptide dataset: 2430 non-binding peptides
The lengthes of the 2430 peptides vary from 8 to 11. The numbers of 8-mer, 9-mer, 10-mer, and 11-mer peptides are 23, 2182, 198, and 27 respectively.
- HLA-B*3501 datasets
- Eluted peptidedataset: 210 naturally processed peptides
The lengthes of the 210 peptides vary from 8 to 11. The numbers of 8-mer, 9-mer, 10-mer, and 11-mer peptides are 16, 153, 28, and 13 respectively.
- Binding peptide dataset: 939 binding peptides
The lengthes of the 939 peptides vary from 8 to 11. The numbers of 8-mer, 9-mer, 10-mer, and 11-mer peptides are 25, 732, 93, and 89 respectively.
- Non-binding peptide dataset: 778 non-binding peptides
The lengthes of the 778 peptides vary from 8 to 11. The numbers of 8-mer, 9-mer, 10-mer, and 11-mer peptides are 21, 565, 177, and 15 respectively.
- HLA-B*4403 datasets
- Eluted peptidedataset: 204 naturally processed peptides
The lengthes of the 204 peptides vary from 8 to 11. The numbers of 8-mer, 9-mer, 10-mer, and 11-mer peptides are 3, 85, 74, and 42 respectively.
- Binding peptide dataset: 218 binding peptides
The lengthes of the 218 peptides vary from 8 to 11. The numbers of 8-mer, 9-mer, 10-mer, and 11-mer peptides are 4, 95, 118, and 1 respectively.
- Non-binding peptide dataset: 321 non-binding peptides
The lengthes of the 321 peptides vary from 8 to 11. The numbers of 8-mer, 9-mer, 10-mer, and 11-mer peptides are 3, 171, 131, and 16 respectively.
- HLA-B*5301 datasets
- Eluted peptidedataset: 121 naturally processed peptides
The lengthes of the 121 peptides vary from 8 to 11.The numbers of 8-mer, 9-mer, 10-mer, and 11-mer, peptides are 16, 84, 20, and 1 respectively.
- Binding peptide dataset: 325 binding peptides
The lengthes of the 325 peptides vary from 8 to 11. The numbers of 8-mer, 9-mer, 10-mer, and 11-mer peptides are 3, 246, 73, and 3 respectively.
- Non-binding peptide dataset: 481 non-binding peptides
The lengthes of the 321 peptides vary from 8 to 11. The numbers of 8-mer, 9-mer, 10-mer, and 11-mer peptides are 25, 252, 182, and 22 respectively.
- HLA-B*5701 datasets
- Eluted peptidedataset: 237 naturally processed peptides
The lengthes of the 237 peptides vary from 8 to 11. The numbers of 8-mer, 9-mer, 10-mer, and 11-mer, peptides are 8, 133, 56, and 40 respectively.
- Binding peptide dataset: 299 binding peptides
The lengthes of the 299 peptides are 9 and 11. The numbers of 9-mer and 11-mer peptides are 287 and 12 respectively.
- Non-binding peptide dataset: 1583 non-binding peptides
The lengthes of the 1583 peptides vary from 9 to 10. The numbers of 9-mer and 10-mer peptides are 1582 and 1 respectively.
- H2-Db datasets
- Eluted peptidedataset: 755 naturally processed peptides
The lengthes of the 755 peptides vary from 8 to 11. The numbers of 8-mer, 9-mer, 10-mer, and 11-mer peptides are 100, 489, 110, and 56 respectively.
- Binding peptide dataset: 891 binding peptides
The lengthes of the 891 peptides vary from 8 to 11. The numbers of 8-mer, 9-mer, 10-mer, and 11-mer peptides are 31, 630, 169, and 61 respectively.
- Non-binding peptide dataset: 1485 non-binding peptides
The lengthes of the 1485 peptides vary from 8 to 11. The numbers of 8-mer, 9-mer, 10-mer, and 11-mer peptides are 305, 612, 384, and 184 respectively.
- H2-Kb datasets
- Eluted peptidedataset: 586 naturally processed peptides
The lengthes of the 586 peptides vary from 8 to 11. The numbers of 8-mer, 9-mer, 10-mer, and 11-mer peptides are 466, 112, 7, and 1 respectively.
- Binding peptide dataset: 1274 binding peptides
The lengthes of the 1274 peptides vary from 8 to 11. The numbers of 8-mer, 9-mer, 10-mer, and 11-mer peptides are 686, 438, 95, and 55 respectively.
- Non-binding peptide dataset: 1324 non-binding peptides
The lengthes of the 1324 peptides vary from 8 to 11. The numbers of 8-mer, 9-mer, 10-mer, and 11-mer peptides are 580, 393, 238, and 113 respectively.
Test data sets with elution properties included:
- HLA-A*0201 datasets
The lengthes of the 1251 peptides vary from 8 to 11. The numbers of 8-mer, 9-mer, 10-mer, and 11-mer peptides are 77, 492, 284, and 398 respectively.
- HLA-B*0702 datasets
The lengthes of the 1727 peptides vary from 8 to 11. The numbers of 8-mer, 9-mer, 10-mer, and 11-mer peptides are 815, 851, 58, and 3 respectively.
- HLA-B*3501 datasets
The lengthes of the 423 peptides vary from 8 to 11. The numbers of 8-mer, 9-mer, 10-mer, and 11-mer peptides are 178, 186, 32, and 27 respectively.
- HLA-B*4403 datasets
The lengthes of the 720 peptides vary from 8 to 10. The numbers of 8-mer, 9-mer, and 10-mer peptides are 330, 335, and 55 respectively.
- HLA-B*5301 datasets
The lengthes of the 485 peptides vary from 8 to 11. The numbers of 8-mer, 9-mer, 10-mer, and 11-mer peptides are 15, 402, 66, and 2 respectively.
- HLA-B*5701 datasets
The lengthes of the 836 peptides vary from 8 to 11. The numbers of 8-mer, 9-mer, 10-mer, and 11-mer peptides are 395, 265, 126, and 50 respectively.
- H2-Db datasets
The lengthes of the 1685 peptides vary from 8 to 11. The numbers of 8-mer, 9-mer, 10-mer, and 11-mer peptides are 297, 920, 363, and 105 respectively.
- H2-Kb datasets
The lengthes of the 1848 peptides vary from 8 to 10. The numbers of 8-mer, 9-mer, and 10-mer peptides are 997, 664, and 187 respectively.
|